Two hep-th papers are "conceptual" today. First, Eva Silverstein wrote her report from the December 2015 German meeting with philosophers "Why Trust a Theory",
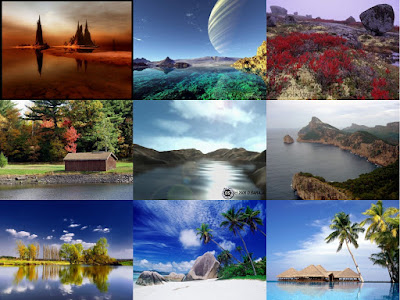
Yang-Hui He (London, Oxford, Tianjin) wrote something fascinating,
The objects that He is classifying including various Calabi-Yau topologies – complete intersections, both 3-folds and 4-folds, hypersurfaces in projective spaces, Calabi-Yaus from reflexive polytopes, vector bundles in the heterotic string, quivers producing gauge theories, and a few others.
The questions he wants to classify or ask about the objects are how many generations there are, whether the Hodge numbers are low or high, and so on.
The databases he is applying the methodology to contain from thousands to ten billion objects. In principle, you could imagine bigger numbers. Some people have said that it's a hopeless task to get familiar with the properties of a large number of compactifications, and that's why "it's not science". Except that these mammals are full of šit. He just places the problem in Mathematica, lets it work for one minute, and then it's enough to read the results. For example, in 96% of some new Calabi-Yaus, the computer correctly says whether the compactification has a high number of complex parameters. Or after a training based on 40% (3,000) compactifications, the computer is capable of correctly guessing the exact value of \(h^{2,1}\) for 80% of the remaining compactifications!
It's plausible that sometime in the future, perhaps in 2030, perhaps tonight, He will run a command like: Please, Wolfram Mathematica,
Even if you describe the compactifications incompletely – if you only talk about some classes that are further divided according to fluxes etc. – it's plausible that a machine learning software sees the classes of compactifications that are by far more promising than others, or something like that, so the information describing the correct compactification could be found by identifying the correct answers to one question after another.
He already has a guess where the phenomenologically relevant compactification is located: it is a desirable residence within a barren vista. If you use Google Street View on top of Wolfram Mathematica, you may find out that it is here in Las Vegas. ;-) By the way, I think that He is misinterpreting what the swampland means when he writes "hints have emerged that the vastness of the landscape might well be mostly a swamp-land". My understanding is that Vafa's swampland is by definition disjoint with the string landscape.
The dangerous irrelevance of string theory"Dangerous irrelevance" isn't a special example of "irrelevance" in the colloquial sense. Instead, it's the dependence of physics on the laws that are valid at "higher than probed" energy scales. She discusses some totally standard technical questions that are being settled by the ongoing research of string cosmology – and explains why this research is an unquestionable example of the scientific method even when it comes to the seemingly most abstract questions such as the "existence of the landscape".
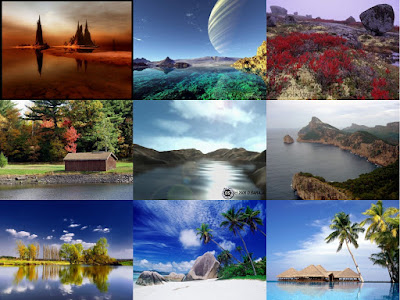
Yang-Hui He (London, Oxford, Tianjin) wrote something fascinating,
Deep-Learning the LandscapeHe uses Wolfram Mathematica (the word "He" is correct even if the author is female! But in this case, he is not) to turn a computer to a hard-working string theorist. I've been calling for such advances for years but he actually did it. Using the machine learning functions, He can train His PC to find new patterns (methods to classify) in the "landscape data" as well as predict some properties of a compactification.
The objects that He is classifying including various Calabi-Yau topologies – complete intersections, both 3-folds and 4-folds, hypersurfaces in projective spaces, Calabi-Yaus from reflexive polytopes, vector bundles in the heterotic string, quivers producing gauge theories, and a few others.
The questions he wants to classify or ask about the objects are how many generations there are, whether the Hodge numbers are low or high, and so on.
The databases he is applying the methodology to contain from thousands to ten billion objects. In principle, you could imagine bigger numbers. Some people have said that it's a hopeless task to get familiar with the properties of a large number of compactifications, and that's why "it's not science". Except that these mammals are full of šit. He just places the problem in Mathematica, lets it work for one minute, and then it's enough to read the results. For example, in 96% of some new Calabi-Yaus, the computer correctly says whether the compactification has a high number of complex parameters. Or after a training based on 40% (3,000) compactifications, the computer is capable of correctly guessing the exact value of \(h^{2,1}\) for 80% of the remaining compactifications!
It's plausible that sometime in the future, perhaps in 2030, perhaps tonight, He will run a command like: Please, Wolfram Mathematica,
TellMeWhichPaper ["from", "the", "arXiv", {"contains", "the right", "compactification", "for a theory of everything"}]The computer will work for three minutes and then it spits the right theory of everything. Problem solved. ;-) Maybe I am oversimplifying just a little bit. But a computer could be courageous and not being afraid of some – straightforward to see – patterns in a list of thousands or billions of objects.
Even if you describe the compactifications incompletely – if you only talk about some classes that are further divided according to fluxes etc. – it's plausible that a machine learning software sees the classes of compactifications that are by far more promising than others, or something like that, so the information describing the correct compactification could be found by identifying the correct answers to one question after another.
He already has a guess where the phenomenologically relevant compactification is located: it is a desirable residence within a barren vista. If you use Google Street View on top of Wolfram Mathematica, you may find out that it is here in Las Vegas. ;-) By the way, I think that He is misinterpreting what the swampland means when he writes "hints have emerged that the vastness of the landscape might well be mostly a swamp-land". My understanding is that Vafa's swampland is by definition disjoint with the string landscape.
Deep-learning the landscape
Reviewed by DAL
on
June 11, 2017
Rating:
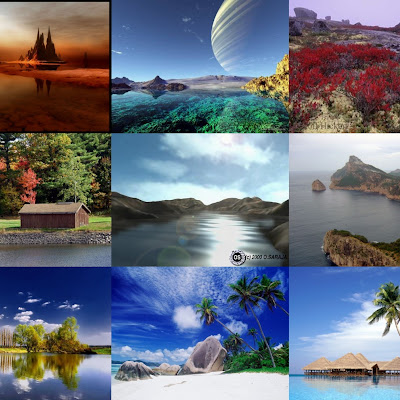
No comments: